Genome wide association
Introduction
We have developed several optimization approaches for Genomewide association study (GWAS) using single nucleotide polymorphism.
.
Exploration of microRNA Genomic Variation Associated with Common Human Diseases.
Joel Fontanarosa and Yang Dai.
Book chapter in microRNAs in Toxicology and Medicine.
Link to Book
Link to PDF
Description
TFor heritable multifactorial diseases, genotypic variation is known to be only one component
of the pathogenesis (Hawkins et al., 2010; Moore et al., 2010). Recently, a number of studies
have sought to analyze the extent to which genomic variation itself may cause modulations
in transcriptional activity via microRNAs to contribute to complex traits or disease
pathogenesis (Martins et al., 201 1; Gamazon et al., 2012a; Gamazon et al., 2012b;
Geeleher et al., 2012; Gong et al., 2012; Slaby et al., 2012; Zhang et al., 2013). Data ……
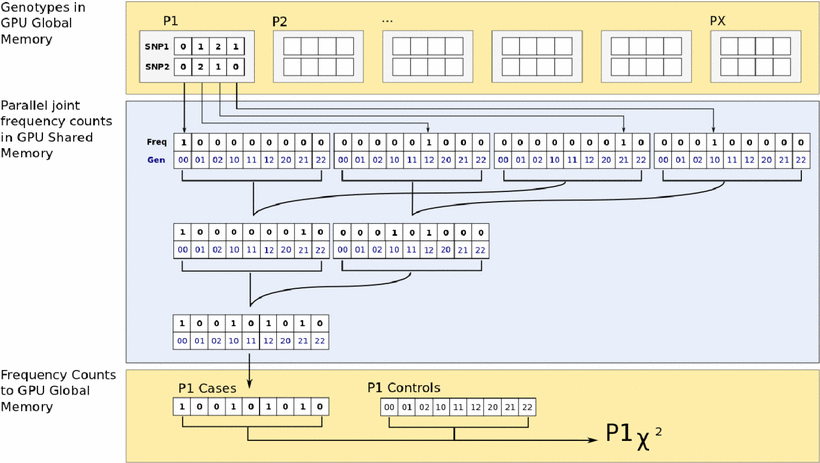
An Evolutionary Optimization Strategy Using Graphics Processing Units to Efficiently Investigate Gene-Gene Interactions in Genetic Association Studies.
Joel Fontanarosa and Yang Dai.
Conf. Proc. IEEE Eng. Med. Biol. Soc. (EMBC 2011) 2011:5547-50. doi: 10.1109/IEMBS.2011.6091415. Link to paper.
Abstract The analysis of gene-gene interactions related to common complex human diseases is complicated by the increasing scale of genetic association analysis. Concurrent with the advances in genetic technology that led to these large data sets, improvements have been made in parallel computing with graphics processing units (GPUs). The data-intensive nature of genetic association analysis makes this problem particularly suitable for improved computation with the powerful computing resources available in GPUs. In this study, we present a GPU-accelerated discrete optimization strategy to improve the computational efficiency of multi-locus association analysis. We implemented an adaptive evolutionary algorithm that takes advantage of linkage disequilibrium to reduce the need for exhaustive search for combinations of genetic markers. The proposed GPU algorithm was shown to have improved efficiency and equivalent power relative to the CPU version.
Using LASSO regression to detect predictive aggregate effects in genetic studies.
Joel Fontanarosa and Yang Dai
BMC Proceedings (2011) 5 (Supple 9): S69. Link to paper
Abstract
We use least absolute shrinkage and selection operator (lasso) regression to select genetic markers and phenotypic features that are most informative with respect to a trait of interest. We compare several strategies for applying lasso methods in risk prediction models, using the Genetic Analysis Workshop 17 exome simulation data consisting of 697 individuals with information on genotypic and phenotypic features (smoking, age, sex) in 5-fold cross-validated fashion. The cross-validated averages of the area under the receiver operating curve range from 0.45 to 0.63 for different strategies using only genotypic markers. The same values are improved to 0.69–0.87 when both genotypic and phenotypic information are used. The ability of the LASSO method to find true causal markers is limited, but the method was able to discover several common variants (e.g., FLT1) under certain conditions.
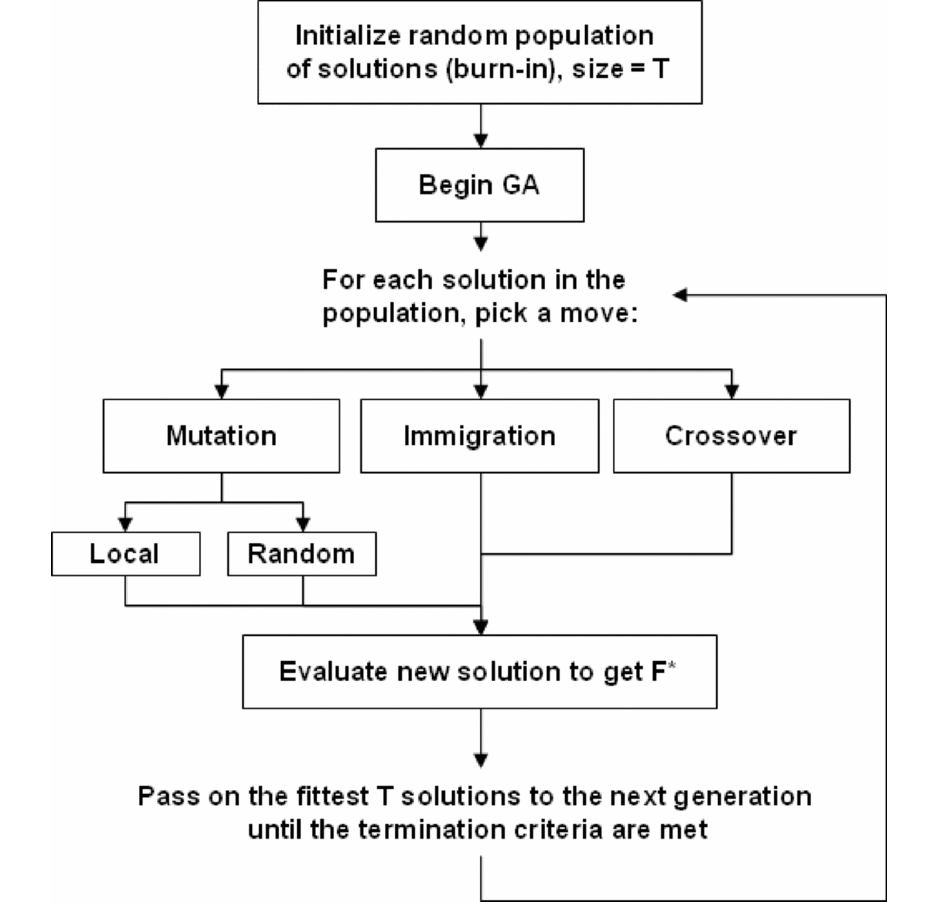
A Block-Based Evolutionary Optimization Strategy to Investigate Gene-Gene Interactions in Genetic Association StudieA Block-Based Evolutionary Optimization Strategy to Investigate Gene-Gene Interactions in Genetic Association Studies.
Joel Fontanarosa and Yang Dai.
Proceedings of 2010 IEEE International conference on Bioinformatics and Biomedicine Workshop, (2010) 330-335
Link to paper.
Abstract
Multi-locus interactions in genetic association studies are believed to influence the heritability of a number of common diseases. In this study, we propose a discrete optimization strategy to improve the power and computational efficiency of multi-locus association analysis. We implemented an adaptive evolutionary algorithm in combination with a linkage disequilibrium-based discretization approach to reduce the need for exhaustive search for combinations by taking advantage of inherent genomic structure. The method was applied to several simulated disease models as well as to a real genome-wide association study. The results indicate that our method performs as well as or better than the most powerful competing methods for detecting true interactions, and it achieves this performance with improved computational efficiency.
Selection of Multiple SNPs in Case-Control Association Study Based on a Discretized Network Flow Approach.
Shantanu Dutt, Yang Dai, Huan Ren and Joel Fontanarosa.
Proceedings of the First Conference of Bioinformatics and Computational Biology (BICoB 2009)
Lecture Notes in Computer Science, Springer Verlag, Vol. 5462, pp.1611-3349, 2009.
Link to PDF.
Abstract
Recent large scale genome-wide association studies have been considered to hold promise for unraveling the genetic etiology of complex diseases. It becomes possible now to use these data to assess the influence of interactions from multiple SNPs on a disease. In this paper we formulate the multiple SNP selection problem for determining genetic risk profiles of certain diseases by formulating novel 0/1 IP formulations for this problem, and solving them using a new near-optimal and efficient discrete optimization technique called discretized network flow that has recently been developed by us. One of the highlights of our approach to solving the multiple SNP selection problem is recognizing that there could be different genetic profiles of a disease among the patient population, and it is thus desirable to classify/cluster patients with similar genetic profiles of the disease while simultaneously selecting the right genetic marker sets of the disease for each cluster. This approach coupled with the DNF technique has yielded results for several diseases with some of the highest sensitivities seen so far and specificities that are higher or comparable to state-of-the art techniques, at a fraction of the runtime of these techniques.