DNA methylation analysis and Enhancer prediction using DNA methylation and histone modification profiles
Introduction
body{ background-color: #ccccff;}
We developed computation methods for prediction of human enhancer based on whole genome DNA methylation profiles
MeDEStrand: an improved method to infer genome-wide absolute methylation levels from DNA enrichment data
Xu J, Liu S, Yin P, Bulun S, and Dai Y.
BMC Bioinformatics (2018), 19(1):540. DOI: 10.1186/s12859-018-2574-7. PPMID: 30577750
PubMed
Abstract
BACKGROUND:
DNA methylation of CpG dinucleotides is an essential epigenetic modification that plays a key role in transcription. Widely used DNA enrichment-based methods offer high coverage for measuring methylated CpG dinucleotides, with the lowest cost per CpG covered genome-wide. However, these methods measure the DNA enrichment of methyl-CpG binding, and thus do not provide information on absolute methylation levels. Further, the enrichment is influenced by various confounding factors in addition to methylation status, for example, CpG density. Computational models that can accurately derive absolute methylation levels from DNA enrichment data are needed.
RESULTS:
We developed “MeDEStrand,” a method that uses a sigmoid function to estimate and correct the CpG bias from enrichment results to infer absolute DNA methylation levels. Unlike previous methods, which estimate CpG bias based on reads mapped at the same genomic loci, MeDEStrand processes the reads for the positive and negative DNA strands separately. We compared the performance of MeDEStrand to that of three other state-of-the-art methods “MEDIPS,” “BayMeth,” and “QSEA” on four independent datasets generated using immortalized cell lines (GM12878 and K562) and human primary cells (foreskin fibroblasts and mammary epithelial cells). Based on the comparison of the inferred absolute methylation levels from MeDIP-seq data and the corresponding reduced-representation bisulfite sequencing data from each method, MeDEStrand showed the best performance at high resolution of 25, 50, and 100 base pairs.
CONCLUSIONS:
The MeDEStrand tool can be used to infer whole-genome absolute DNA methylation levels at the same cost of enrichment-based methods with adequate accuracy and resolution. R package MeDEStrand and its tutorial are freely available for download at https://github.com/jxu1234/MeDEStrand.git .
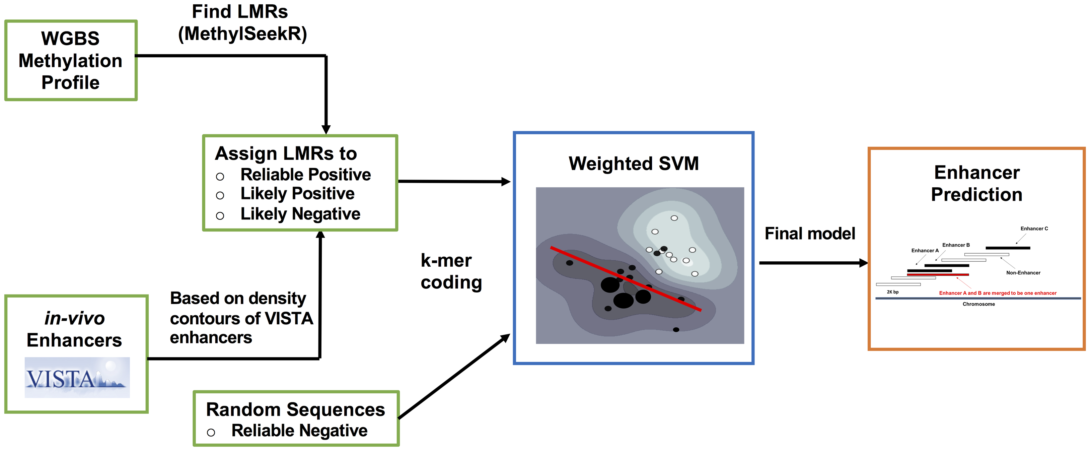
LMethyR-SVM: Predict Human Enhancers Using Low Methylated Regions based on Weighted Support Vector Machines
Xu J, Hu H, Dai Y.
PLoS ONE, (2016) 11(9): e0163491. doi:10.1371/journal.pone.0163491. PMID: 27662487.
PubMed
Abstract
Background The identification of enhancers is a challenging task. Various types of epigenetic information including histone modification have been utilized in the construction of enhancer prediction models based on a diverse panel of machine learning schemes. However, DNA methylation profiles generated from the whole genome bisulfite sequencing (WGBS) have not been fully explored for their potential in enhancer prediction despite the fact that low methylated regions (LMRs) have been implied to be distal active regulatory regions.
Method In this work, we propose a prediction framework, LMethyR-SVM, using LMRs identified from cell-type-specific WGBS DNA methylation profiles and a weighted support vector machine learning framework. In LMethyR-SVM, the set of cell-type-specific LMRs is further divided into three sets: reliable positive, like positive and likely negative, according to their resemblance to a small set of experimentally validated enhancers in the VISTA database based on an estimated non-parametric density distribution. Then, the prediction model is obtained by solving a weighted support vector machine.
Results We demonstrate the performance of LMethyR-SVM by using the WGBS DNA methylation profiles derived from the human embryonic stem cell type (H1) and the fetal lung fibroblast cell type (IMR90). The predicted enhancers are highly conserved with a reasonable validation rate based on a set of commonly used positive markers including transcription factors, p300 binding and DNase-I hypersensitive sites. In addition, we show evidence that the large fraction of the LMethyR-SVM predicted enhancers are not predicted by ChromHMM in H1 cell type and they are more enriched for the FANTOM5 enhancers.