Modeling gene regulation and cellular heterogeneity
Introduction
body { background-color: #F4ECF7;}
We have developed a variety of frameworks for modeling gene regulation and cellular heterogeneity. Bayesian network, support vector machines, LASSO, random forest and other statistical and machine learning models were based on gene expression, microRNA expression, global transcription factor binding and epigenomic profiles (ChIP-seq) and DNA methylation.
A computational approach to identify cellular heterogeneity and tissue-specific gene regulatory networks.
Jambusaria A, Klomp J, Hong Z, Rafii S, Dai Y, Malik AB, Rehman J.
BMC Bioinformatics
(2018) 19(1):217. doi: 10.1186/s12859-018-2190-6.
Link to PubMed.
Abstract
BACKGROUND:
The heterogeneity of cells across tissue types represents a major challenge for studying biological mechanisms as well as for therapeutic targeting of distinct tissues. Computational prediction of tissue-specific gene regulatory networks may provide important insights into the mechanisms underlying the cellular heterogeneity of cells in distinct organs and tissues.
RESULTS:
Using three pathway analysis techniques, gene set enrichment analysis (GSEA), parametric analysis of gene set enrichment (PGSEA), alongside our novel model (HeteroPath), which assesses heterogeneously upregulated and downregulated genes within the context of pathways, we generated distinct tissue-specific gene regulatory networks. We analyzed gene expression data derived from freshly isolated heart, brain, and lung endothelial cells and populations of neurons in the hippocampus, cingulate cortex, and amygdala. In both datasets, we found that HeteroPath segregated the distinct cellular populations by identifying regulatory pathways that were not identified by GSEA or PGSEA. Using simulated datasets, HeteroPath demonstrated robustness that was comparable to what was seen using existing gene set enrichment methods. Furthermore, we generated tissue-specific gene regulatory networks involved in vascular heterogeneity and neuronal heterogeneity by performing motif enrichment of the heterogeneous genes identified by HeteroPath and linking the enriched motifs to regulatory transcription factors in the ENCODE database.
CONCLUSIONS:
HeteroPath assesses contextual bidirectional gene expression within pathways and thus allows for transcriptomic assessment of cellular heterogeneity. Unraveling tissue-specific heterogeneity of gene expression can lead to a better understanding of the molecular underpinnings of tissue-specific phenotypes.
KEYWORDS:
Endothelial cells; Endothelial heterogeneity; Gene expression; Gene set enrichment; Neuronal heterogeneity; Neurons; Pathway analysis; Systems biology; Therapeutic targets; Tissue specificity; Transcription factor binding motifs; Transcriptional networks; Vascular biology
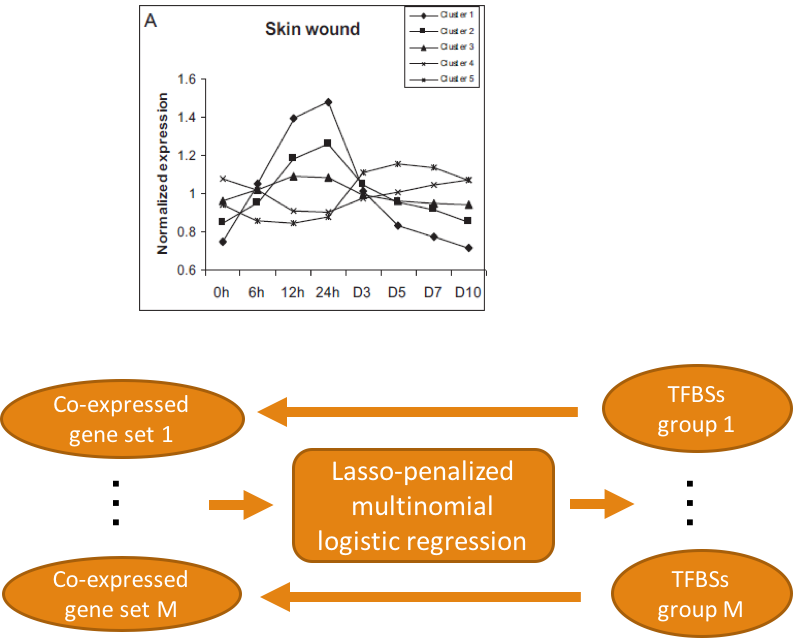
BOOK CHAPTERS:
Prioritize Transcription Factor Binding Sites for Multiple Co-Expressed Gene Sets Based on Lasso Multinomial Regression Models
Hong Hu and Yang Dai
Emerging Research in the Analysis and Modeling of Gene Regulatory Networks. IGI Global (2016) p.280-315.
Link to Abstract.
Abstract
Computational prediction of cis-regulatory elements for a set of co-expressed genes based on sequence analysis provides an overwhelming volume of potential transcription factor binding sites. It presents a challenge to prioritize a set of functional transcription factors and their binding sites on the regulatory regions of the target genes that are relevant to the gene expression study. A novel approach based on the use of lasso multinomial regression models is proposed to address this problem. We examine the ability of the lasso models using a time-course microarray data obtained from a comprehensive study of gene expression profiles in skin and mucosal in mouse over all stages of wound healing.
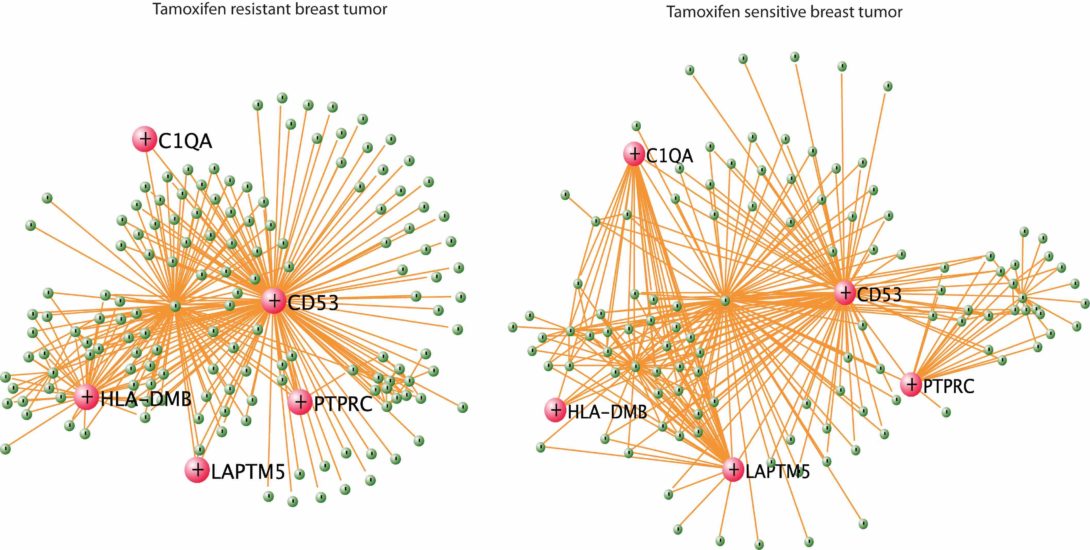
Systems Biology Understanding of Tamoxifen Resistance of Breast Cancer based on Integrative Bioinformatics Approaches,”
Lei Huang and Yang Dai
in Breast Cancer Metastasis and Drug Resistance: Progress and Prospects, (ed. A. Ahmad), Springer-Verlag New York Inc. (2013) pp.249-259. doi:10:100/7/978-1-4614-5647-6_14.
PDF
Abstract
Global gene expression profiles on tumors are not only useful in developing prognosis signatures but also rich resource for the elucidation of underlying mechanisms related to poor clinical outcome and drug resistance. In this chapter we present a panel of bioinformatics strategies to derive biological insights based on gene expression profiles on estrogen receptor positive breast tumors that were collected prior to adjuvant tamoxifen treatment. The analyses reveal that the tamoxifen resistant tumors are highly proliferative and display a distinctive expression profile for genes related to inflammation and angiogenesis compared to tamoxifen sensitive tumors. The bioinformatics analysis also identifies a set of small molecules that may reverse the tamoxifen resistance in breast tumor.
Computational methods for the identification of microRNA targets.
Yang Dai and Xiaofeng Zhou
Open Access Bioinformatics (2010) 2:29-39.
PubMed
Abstract
MicroRNAs (miRNAs) are short RNA molecules that regulate the post-transcriptional expression of their target genes. This regulation may take the form of stable translational or degradation of the target transcript, although the mechanisms governing the outcome of miRNA-mediated regulation remain largely unknown. While it is becoming clear that miRNAs are core components of gene regulatory networks, elucidating precise roles for each miRNA within these networks will require an accurate means of identifying target genes and assessing the impact of miRNAs on individual targets. Numerous computational methods for predicting targets are currently available. These methods vary widely in their emphasis, accuracy, and ease of use for researchers. This review will focus on a comparison of the available computational methods in animals, with an emphasis on approaches that are informed by experimental analysis of microRNA:target complexes.
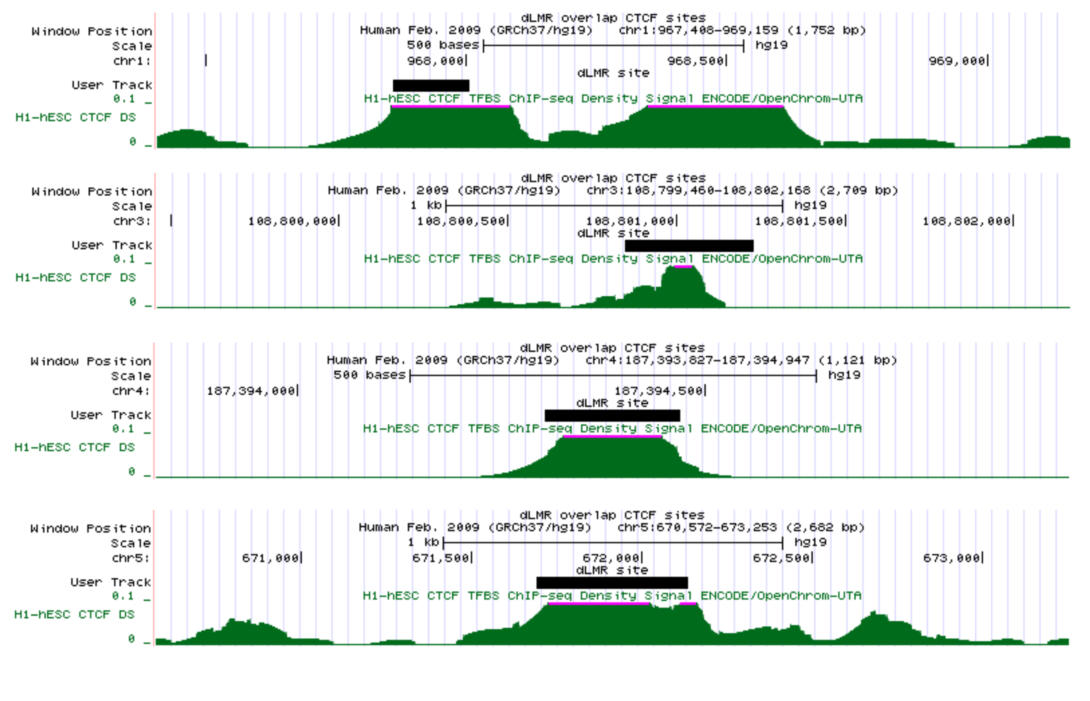
PAPERS:
Regulatory elements in low-methylated regions predict directional change of gene expression
Hong Hu, Jingting Xu and Yang Dai
IEEE J Biomed Health Inform. (2015) Jul;19(4):1293-300. doi: 10.1109/JBHI.2015.2431640.
Link to PubMed.
Abstract
Recent studies on methylomes obtained from the whole genome bisulfite sequencing (WGBS) indicate that low-methylated regions (LMRs) are related to potential active distal regulatory regions such as enhancers in mammalian genomes. To investigate the potential effect of regulatory elements in LMRs on gene expression, we proposed penalized logistic regression models to predict the directional change of differentially expressed genes using predicted transcription factor binding sites (TFBSs) in LMRs that are distinctive between two cell types. We evaluated our models on four cell types where the WGBS and RNA-seq data were available. The average area under the ROC curve (AUC) from the tenfold cross validation was computed over the six pairs of cell types in each model. The models using TFBSs in LMRs in intergenic or genebody region are more predictive (AUC 0.71 and 0.66, respectively) compared with the one using TFBSs from promoter regions alone (AUC 0.62). When using a model that combines TFBSs in LMRs from both intergenic and genebody regions, the best prediction was obtained (AUC 0.78). Our models are capable of identifying subsets of LMRs that are significantly enriched for the ChIP-seq binding sites of the insulator protein CTCF and p300 co-activator and other transcription factors. Our framework provides further evidence of putative distal regulatory elements from LMRs located in intergenic and genebody regions.
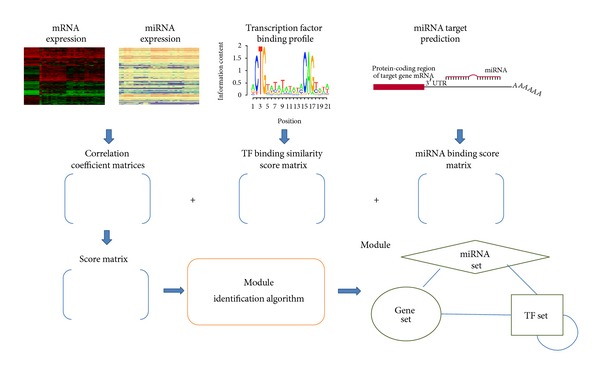
A Local Genetic Algorithm for the Identification of Condition-Specific MicroRNA-Gene Modules.
Wenbo Mu, Damian Roqueiro, Yang Dai
Scientific World Journal (2013). doi:10.1155/2013/197406.
PubMed
Abstract
Transcription factor and microRNA are two types of key regulators of gene expression. Their regulatory mechanisms are highly complex. In this study, we propose a computational method to predict condition-specific regulatory modules that consist of microRNAs, transcription factors, and their commonly regulated genes. We used matched global expression profiles of mRNAs and microRNAs together with the predicted targets of transcription factors and microRNAs to construct an underlying regulatory network. Our method searches for highly scored modules from the network based on a two-step heuristic method that combines genetic and local search algorithms. Using two matched expression datasets, we demonstrate that our method can identify highly scored modules with statistical significance and biological relevance. The identified regulatory modules may provide useful insights on the mechanisms of transcription factors and microRNAs.
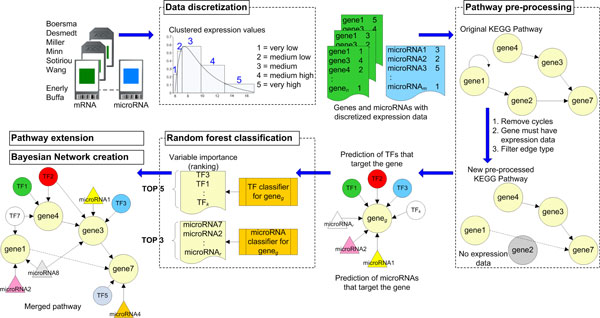
Identifying transcription factors and microRNAs as key regulators of pathways using Bayesian inference on known pathway structures.
Damian Roqueiro, Lei Huang and Yang Dai
Proteome Sci. (2012) 10(Suppl 1): S15. doi: 10.1186/1477-5956-10-S1-S15.
Link to PubMed.
Abstract
Background Transcription factors and microRNAs act in concert to regulate gene expression in eukaryotes. Numerous computational methods based on sequence information are available for the prediction of target genes of transcription factors and microRNAs. Although these methods provide a static snapshot of how genes may be regulated, they are not effective for the identification of condition-specific regulators.
Results We propose a new method that combines: a) transcription factors and microRNAs that are predicted to target genes in pathways, with b) microarray expression profiles of microRNAs and mRNAs, in conjunction with c) the known structure of molecular pathways. These elements are integrated into a Bayesian network derived from each pathway that, through probability inference, allows for the prediction of the key regulators in the pathway. We demonstrate 1) the steps to discretize the expression data for the computation of conditional probabilities in a Bayesian network, 2) the procedure to construct a Bayesian network using the structure of a known pathway and the transcription factors and microRNAs predicted to target genes in that pathway, and 3) the inference results as potential regulators of three signaling pathways using microarray expression profiles of microRNA and mRNA in estrogen receptor positive and estrogen receptor negative tumors.
Conclusions We displayed the ability of our framework to integrate multiple sets of microRNA and mRNA expression data, from two phenotypes, with curated molecular pathway structures by creating Bayesian networks. Moreover, by performing inference on the network using known evidence, e.g., status of differentially expressed genes, or by entering hypotheses to be tested, we obtain a list of potential regulators of the pathways. This, in turn, will help increase our understanding about the regulatory mechanisms relevant to the two phenotypes.
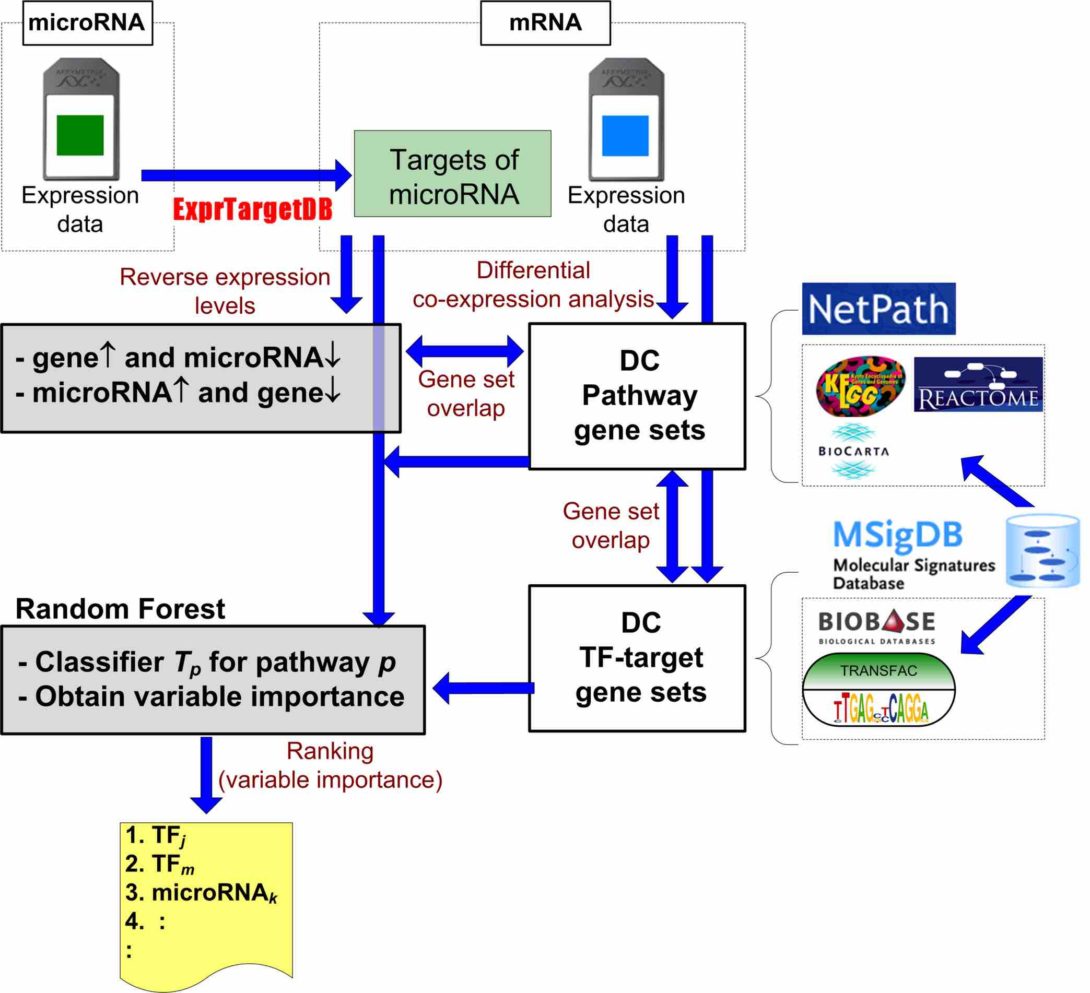
Analyzing mRNA and microRNA co-expression profiles to identify pathways and their potential regulators in ER+ and ER- breast tumors
Lei Huang, Damian Roqueiro and Yang Dai
Conf. Proc. IEEE Eng. Med. Biol. Soc. (EMBC 2011)
Abstract Transcription factors and microRNAs are both considered pivotal regulators of gene expression. Numerous computational methods have been developed to predict their targets. These methods, although powerful, provide a static snapshot of how genes may be regulated by transcription factors and microRNAs. We propose a method that combines these prediction data with co-expression analysis and a supervised learning algorithm to determine the main regulators in different pathways of ER+ and ER- tumors.
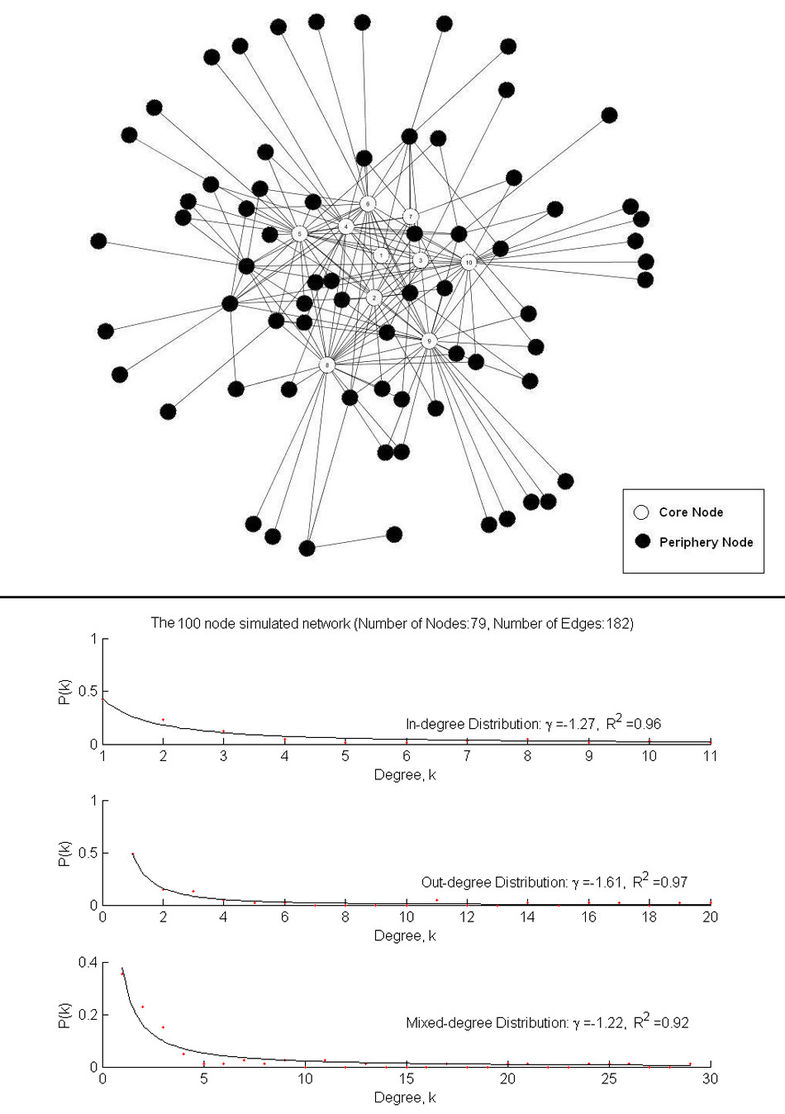
Rank-based edge reconstruction for scale-free genetic regulatory networks
Guanrao Chen, Peter Larsen, Eyad Almasri, Yang Dai
BMC Bioinformatics (2008) 9:75.
Link to PubMed
Abstract
Background The reconstruction of genetic regulatory networks from microarray gene expression data has been a challenging task in bioinformatics. Various approaches to this problem have been proposed, however, they do not take into account the topological characteristics of the targeted networks while reconstructing them.
Results In this study, an algorithm that explores the scale-free topology of networks was proposed based on the modification of a rank-based algorithm for network reconstruction. The new algorithm was evaluated with the use of both simulated and microarray gene expression data. The results demonstrated that the proposed algorithm outperforms the original rank-based algorithm. In addition, in comparison with the Bayesian Network approach, the results show that the proposed algorithm gives much better recovery of the underlying network when sample size is much smaller relative to the number of genes.
Conclusions The proposed algorithm is expected to be useful in the reconstruction of biological networks whose degree distributions follow the scale-free topology.
Incorporating Knowledge of Topology Improves Reconstruction of Interaction Networks from Microarray Data.
Peter Larsen, Eyad Almasri, Guanrao Chen and Yang Dai
Lecture Notes in Bioinformatics (2008) Vol. 4983 (eds.by I.I. Mandoiu, Raj Sunderraman, and A. Zelikovsky), Springer Verlag, pp. 434-443.
Link to Abstract.
Abstract
Reconstruction of biological interaction networks from highthroughput experimental data is one of the most challenging problems in bioinformatics. These networks have specific topologies, whose characteristics are defined by evolutionary relationships between proteins and the physical limitations imposed on proteins interacting in three-dimensional space. In this study, a method is proposed applying the topology of known biological networks to the analysis of microarray data for protein-protein binding interactions. In this method, genomic biological networks are derived from the body of published scientific literature. The numbers of interacting neighbors for proteins of specific molecular functions are observed. That information is used in the analysis of microarray expression data to regenerate biological networks using a rank-based algorithm, Gene Ontology Restricted Value Neighborhood (GRV-N). The results of this analysis demonstrate that incorporating knowledge of network topology improves the ability of expression analysis to reconstruct interaction networks with a high degree of biological relevance.
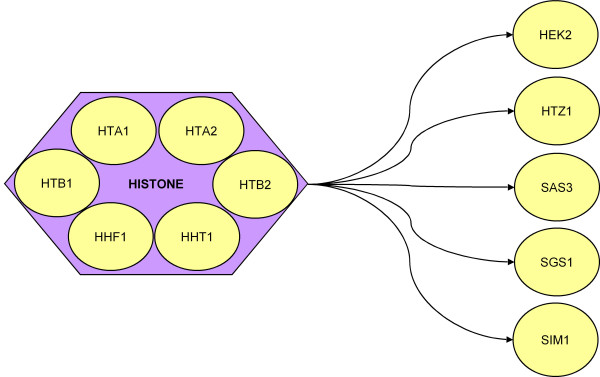
A statistical method to incorporate biological knowledge for generating testable novel gene regulatory interactions from microarray experiments.
Peter Larsen, Eyad Almasri, Guanrao Chen, Yang Dai
BMC Bioinformatics (2007) 8:317.
Link to PubMed.
Abstract
Background The incorporation of prior biological knowledge in the analysis of microarray data has become important in the reconstruction of transcription regulatory networks in a cell. Most of the current research has been focused on the integration of multiple sets of microarray data as well as curated databases for a genome scale reconstruction. However, individual researchers are more interested in the extraction of most useful information from the data of their hypothesis-driven microarray experiments. How to compile the prior biological knowledge from literature to facilitate new hypothesis generation from a microarray experiment is the focus of this work. We propose a novel method based on the statistical analysis of reported gene interactions in PubMed literature.
Results Using Gene Ontology (GO) Molecular Function annotation for reported gene regulatory interactions in PubMed literature, a statistical analysis method was proposed for the derivation of a likelihood of interaction (LOI) score for a pair of genes. The LOI-score and the Pearson correlation coefficient of gene profiles were utilized to check if a pair of query genes would be in the above specified interaction. The method was validated in the analysis of two gene sets formed from the yeast Saccharomyces cerevisiae cell cycle microarray data. It was found that high percentage of identified interactions shares GO Biological Process annotations (39.5% for a 102 interaction enriched gene set and 23.0% for a larger 999 cyclically expressed gene set).
Conclusions This method can uncover novel biologically relevant gene interactions. With stringent confidence levels, small interaction networks can be identified for further establishment of a hypothesis testable by biological experiment. This procedure is computationally inexpensive and can be used as a preprocessing procedure for screening potential biologically relevant gene pairs subject to the analysis with sophisticated statistical methods.