Modeling Host-microbiome Interaction
Advances in metagenomics sequencing, environmental proteomics and metabolomics have enabled researchers to peer into previously invisible ecosystems of prokaryotes, eukaryotes, and viruses. The influx of data has created a wealth of opportunity for novel trans-omics computational and informatics approaches to viewing the living world and to predict how microbiome communities affect their hosts. We present our recent results in analyzing, modeling, and understanding host-microbiome interactions.
These methods involve machine learning methods (Support Vector Machines, Convolution neural networks and other artificial Nerural Networks) to Predict host health status based on the community taxonomical and community functional profiles derived gut microbiomePredict ecological niche of bacteria.
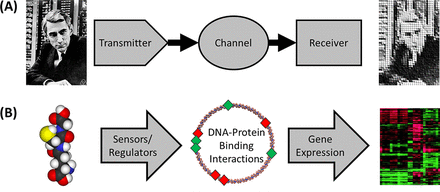
Modeling the Pseudomonas Sulfur Regulome by Quantifying the Storage and Communication of Information
Larsen PE, Zerbs S, Laible PD, Collart FR, Korajczyk P, Dai Y, and Noirot.P.
mSystems. 2018;3(3).
Link to PubMed.
Abstract
Bacteria are not simply passive consumers of nutrients or merely steady-state systems. Rather, bacteria are active participants in their environments, collecting information from their surroundings and processing and using that information to adapt their behavior and optimize survival. The bacterial regulome is the set of physical interactions that link environmental information to the expression of genes by way of networks of sensors, transporters, signal cascades, and transcription factors. As bacteria cannot have one dedicated sensor and regulatory response system for every possible condition that they may encounter, the sensor systems must respond to a variety of overlapping stimuli and collate multiple forms of information to make “decisions” about the most appropriate response to a specific set of environmental conditions. Here, we analyze Pseudomonas fluorescens transcriptional responses to multiple sulfur nutrient sources to generate a predictive, computational model of the sulfur regulome. To model the regulome, we utilize a transmitter-channel-receiver scheme of information transfer and utilize principles from information theory to portray P. fluorescens as an informatics system. This approach enables us to exploit the well-established metrics associated with information theory to model the sulfur regulome. Our computational modeling analysis results in the accurate prediction of gene expression patterns in response to the specific sulfur nutrient environments and provides insights into the molecular mechanisms of Pseudomonas sensory capabilities and gene regulatory networks. In addition, modeling the bacterial regulome using the tools of information theory is a powerful and generalizable approach that will have multiple future applications to other bacterial regulomes. IMPORTANCE Bacteria sense and respond to their environments using a sophisticated array of sensors and regulatory networks to optimize their fitness and survival in a constantly changing environment. Understanding how these regulatory and sensory networks work will provide the capacity to predict bacterial behaviors and, potentially, to manipulate their interactions with an environment or host. Leveraging the information theory provides useful quantitative metrics for modeling the information processing capacity of bacterial regulatory networks. As our model accurately predicted gene expression profiles in a bacterial model system, we posit that the information theory-based approaches will be important to enhance our understanding of a wide variety of bacterial regulomes and our ability to engineer bacterial sensory and regulatory networks.
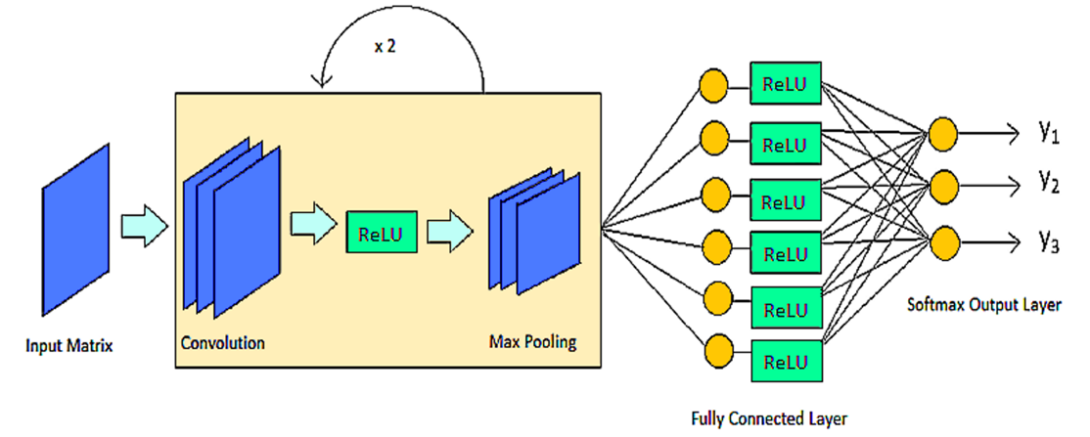
Using Convolutional Neural Networks to Explore the Microbiome
Derek Reiman, Ahmed Metwally and Yang Dai
Proc. of the Annual International Conference of the IEEE (2017)
Link to IEEE Explore.
Abstract
The microbiome has been shown to have an impact on the development of various diseases in the host. Being able to make an accurate prediction of the phenotype of a genomic sample based on its microbial taxonomic abundance profile is an important problem for personalized medicine. In this paper, we examine the potential of using a deep learning framework, a convolutional neural network (CNN), for such a prediction. To facilitate the CNN learning, we explore the structure of abundance profiles by creating the phylogenetic tree and by designing a scheme to embed the tree to a matrix that retains the spatial relationship of nodes in the tree and their quantitative characteristics. The proposed CNN framework is highly accurate, achieving a 99.47% of accuracy based on the evaluation on a dataset 1967 samples of three phenotypes. Our result demonstrated the feasibility and promising aspect of CNN in the classification of sample phylotype.
We have received a GPU Grant (a TITAN Xp GPU Unit) from NVIDIA to develop efficent algorithms to train Converlution Neural Networks (CNN) for the prediction of phenotypes of microbiome samples and for identification of important features representing the samples.
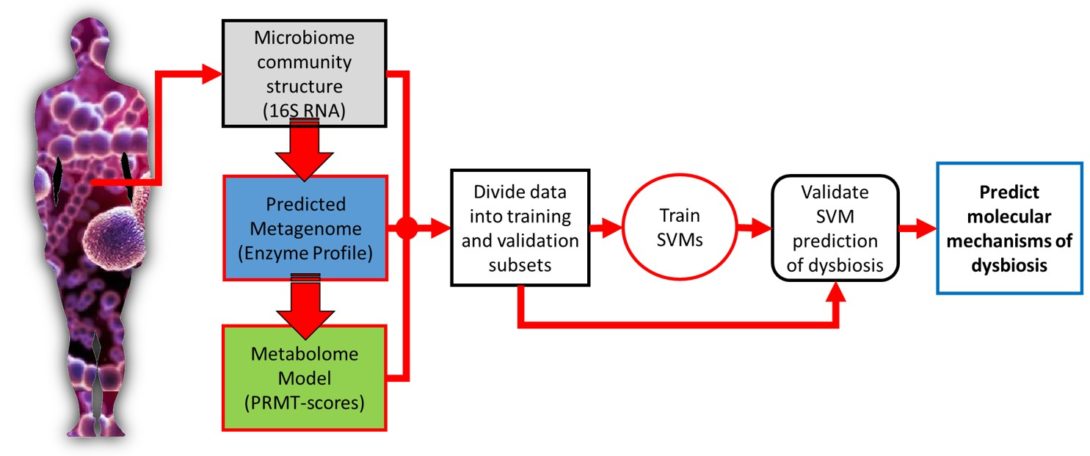
Metabolome of human gut microbiome is predictive of host dysbiosis
Peter Larsen and Yang Dai
GigaScience (2015) 4 (1) pp.42. DOI:10.1186/s13742-015-0084-3. PMID: 26380076.
PubMed.
Abstract
BACKGROUND: Humans live in constant and vital symbiosis with a closely linked bacterial ecosystem called the microbiome, which influences many aspects of human health. When this microbial ecosystem becomes disrupted, the health of the human host can suffer; a condition called dysbiosis. However, the community compositions of human microbiomes also vary dramatically from individual to individual, and over time, making it difficult to uncover the underlying mechanisms linking the microbiome to human health. We propose that a microbiome’s interaction with its human host is not necessarily dependent upon the presence or absence of particular bacterial species, but instead is dependent on its community metabolome; an emergent property of the microbiome.
RESULTS: Using data from a previously published, longitudinal study of microbiome populations of the human gut, we extrapolated information about microbiome community enzyme profiles and metabolome models. Using machine learning techniques, we demonstrated that the aggregate predicted community enzyme function profiles and modeled metabolomes of a microbiome are more predictive of dysbiosis than either observed microbiome community composition or predicted enzyme function profiles.
CONCLUSIONS: Specific enzyme functions and metabolites predictive of dysbiosis provide insights into the molecular mechanisms of microbiome-host interactions. The ability to use machine learning to predict dysbiosis from microbiome community interaction data provides a potentially powerful tool for understanding the links between the human microbiome and human health, pointing to potential microbiome-based diagnostics and therapeutic interventions.
KEYWORDS: Dysbiosis; Gut microbiome; Human microbiome; Machine learning; Metabolome modeling; Metagenomics; Microbial communities
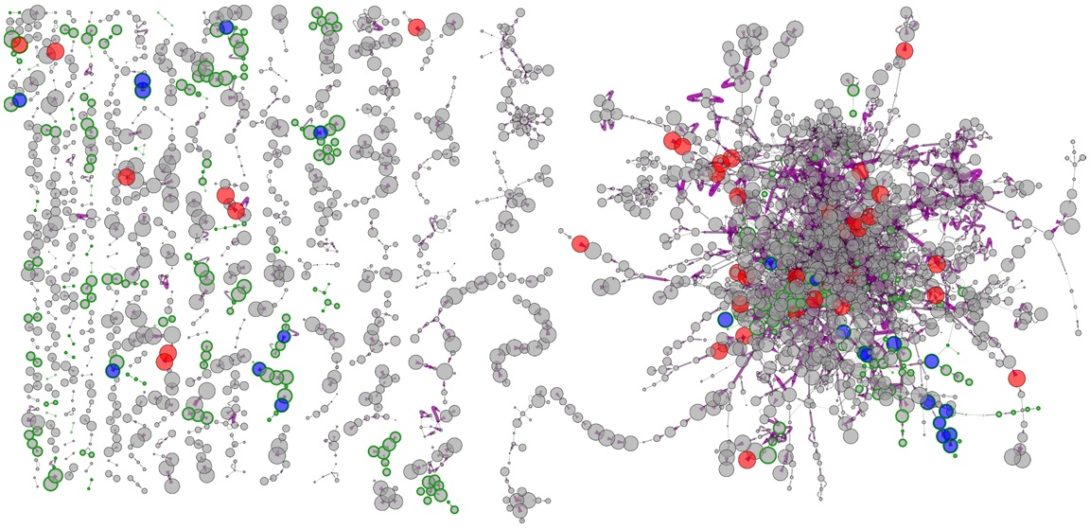
Multi-Omics Approach Identifies Molecular Mechanisms of Plant-Fungus Mycorrhizal Interaction.
Peter E. Larsen, Avinash Sreedasyam, Geetika Trivedi, Shalaka Desai, Yang Dai, Leland J. Cseke, and Frank R. Collart
Frontiers in Plant Science, (2016) 1061. DOI: 10.3389/fpls.2015.01061. PMC4717292.
Link to PubMed.
Abstract
In mycorrhizal symbiosis, plant roots form close, mutually beneficial interactions with soil fungi. Before this mycorrhizal interaction can be established however, plant roots must be capable of detecting potential beneficial fungal partners and initiating the gene expression patterns necessary to begin symbiosis. To predict a plant root—mycorrhizal fungi sensor systems, we analyzed in vitro experiments of Populus tremuloides (aspen tree) and Laccaria bicolor (mycorrhizal fungi) interaction and leveraged over 200 previously published transcriptomic experimental data sets, 159 experimentally validated plant transcription factor binding motifs, and more than 120-thousand experimentally validated protein-protein interactions to generate models of pre-mycorrhizal sensor systems in aspen root. These sensor mechanisms link extracellular signaling molecules with gene regulation through a network comprised of membrane receptors, signal cascade proteins, transcription factors, and transcription factor binding DNA motifs. Modeling predicted four pre-mycorrhizal sensor complexes in aspen that interact with 15 transcription factors to regulate the expression of 1184 genes in response to extracellular signals synthesized by Laccaria. Predicted extracellular signaling molecules include common signaling molecules such as phenylpropanoids, salicylate, and jasmonic acid. This multi-omic computational modeling approach for predicting the complex sensory networks yielded specific, testable biological hypotheses for mycorrhizal interaction signaling compounds, sensor complexes, and mechanisms of gene regulation.
Keywords: Laccaria bicolor, Populus tremuloides, mycorrhizae, metabolomics, transcriptomics, proteomics, system modeling
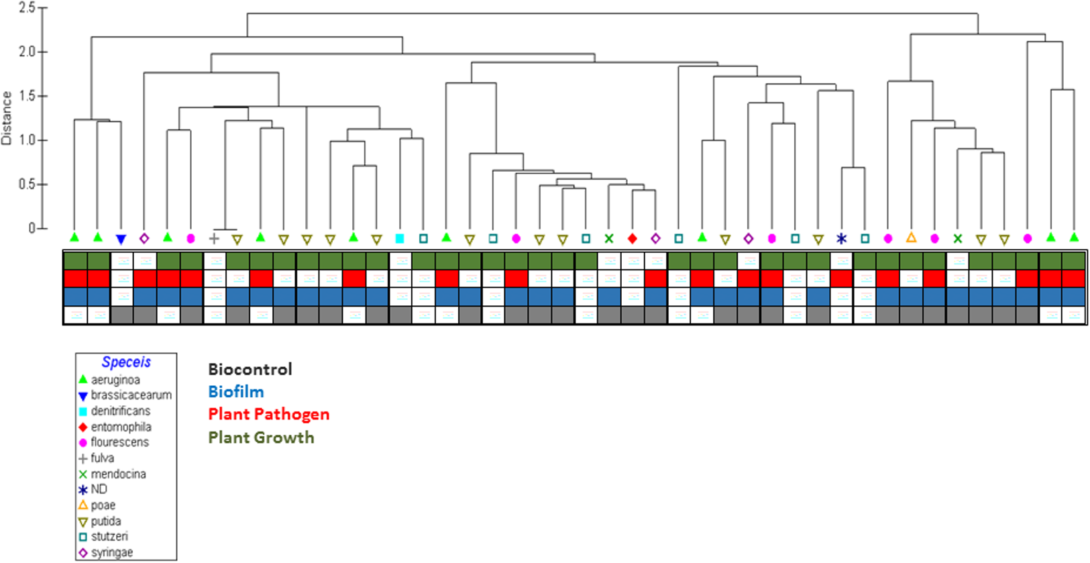
Predicting Ecological Roles in the Rhizosphere Using Metabolome and Transportome Modeling.
Peter Larsen, Frank Collart and Yang Dai
PLoS ONE (2015) 10 (9), e0132837. DOI: 10.1371/journal.pone.0132837. PMID: 26332409.
Link to PubMed.
Abstract
The ability to obtain complete genome sequences from bacteria in environmental samples, such as soil samples from the rhizosphere, has highlighted the microbial diversity and complexity of environmental communities. However, new algorithms to analyze genome sequence information in the context of community structure are needed to enhance our understanding of the specific ecological roles of these organisms in soil environments. We present a machine learning approach using sequenced Pseudomonad genomes coupled with outputs of metabolic and transportomic computational models for identifying the most predictive molecular mechanisms indicative of a Pseudomonad’s ecological role in the rhizosphere: a biofilm, biocontrol agent, promoter of plant growth, or plant pathogen. Computational predictions of ecological niche were highly accurate overall with models trained on transportomic model output being the most accurate (Leave One Out Validation F-scores between 0.82 and 0.89). The strongest predictive molecular mechanism features for rhizosphere ecological niche overlap with many previously reported analyses of Pseudomonad interactions in the rhizosphere, suggesting that this approach successfully informs a system-scale level understanding of how Pseudomonads sense and interact with their environments. The observation that an organism’s transportome is highly predictive of its ecological niche is a novel discovery and may have implications in our understanding microbial ecology. The framework developed here can be generalized to the analysis of any bacteria across a wide range of environments and ecological niches making this approach a powerful tool for providing insights into functional predictions from bacterial genomic data.
Using metabolomic and transportomic modeling and machine learning to identify putative novel therapeutic targets for antibiotic resistant Pseudomonad infections
Peter Larsen, Frank Collart and Yang Dai
Proc. of the Annual International Conference of the IEEE (2014) pp.314-317. doi: 10.1109/EMBC.2014.6943592. PMID: 25569960.
Link to IEEE Explore.
Abstract
Hospital acquired infections sicken or kill tens of thousands of patients every year. These infections are difficult to treat due to a growing prevalence of resistance to many antibiotics. Among these hospital acquired infections, bacteria of the genus Pseudomonas are among the most common opportunistic pathogens. Computational methods for predicting potential novel antimicrobial therapies for hospital acquired Pseudomonad infections, as well as other hospital acquired infectious pathogens, are desperately needed. Using data generated from sequenced Pseudomonad genomes and metabolomic and transportomic computational approaches developed in our laboratory, we present a support vector machine learning method for identifying the most predictive molecular mechanisms that distinguish pathogenic from non-pathogenic Pseudomonads. Predictions were highly accurate, yielding F-scores between 0.84 and 0.98 in leave one out cross validations. These mechanisms are high-value targets for the development of new antimicrobial therapies.
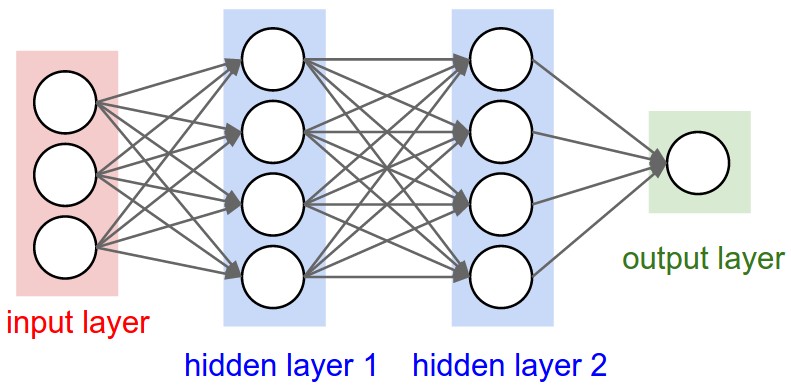
Prediction Bacterial Community Assemblages using an Artificial Neural Network Approach
Peter Larsen, Yang Dai and Frank Collart
in Methods Mol Biol., Springer, (2015) 1260:33-43. doi: 10.1007/978-1-4939-2239-0_3. PMID: 25502374.
Link to PubMed.
Abstract
Microbial communities are found in nearly all environments and play a critical role in defining ecosystem service. Understanding the relationship between these microbial communities and their environment is essential for prediction of community structure, robustness, and response to ecosystem changes. Microbial Assemblage Prediction (MAP) describes microbial community structure as an artificial neural network (ANN) that models the microbial community as functions of environmental parameters and community intra-microbial interactions. MAP models can be used to predict community assemblages over a wide range of possible environmental parameters, extrapolate the results of point observations across spatial scales, and make predictions about how microbial communities may fluctuate as the result of changes in their environment.